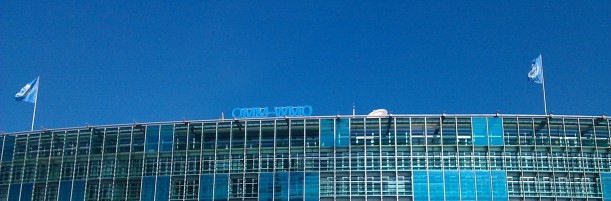
Headquarters of the WMO, which we visited during the conference for a discussion on the socioeconomic benefits of meteorological satellites.
The EUMETSAT Meteorological Satellites Conference 2014 featured a lot of new science. Two particular points which stood out to me was the assimilation of new products into numerical weather forecasting systems, and the use of satellite data in improving our conceptual understanding of weather systems.
Until this conference I was not aware how new it was to incorporate soil moisture into numerical weather forecasting systems. Such forecasting systems spend a good deal of resources on assimilating observational data to initialise the forecast. This is very important because, as pioneering work by Ed Lorenz showed back in the 1960s, tiny differences in the initial state of an atmospheric model (and of course the real atmosphere) can lead to huge differences in the resulting forecast, even for relatively short-range forecasts.
Soil moisture is clearly a useful thing to know about in our forecasts. For weather forecasts it mainly plays a role in supplying water for weather systems. Wet surfaces supply water to the atmosphere, causing or intensifying rainfall.
A few years ago soil moisture satellite products were not considered mature enough to assimilate into weather forecast systems. This is partly because our measurements were quite uncertain (we couldn’t attach very accurate numbers to them), but also because our uncertainty was poorly characterised (we didn’t know how accurate our measurements were). In a sense, the latter is more important. Like much of science, the point is not always knowing things exactly, but accepting that it’s impossible to achieve perfect accuracy and to at least know exactly how certain we are about a measurement (Tamsin Edwards has a related blog post focusing on climate rather than weather).
After some experimental studies showed the potential for soil moisture data to improve weather forecasts, operational forecasting centres across the world began to adopt this extra data source – the ones I heard about at the conference were ECMWF and the UK Met Office, but there are probably others.
Now let’s move to something less mathematical, but equally as important and exciting. On Thursday I listened to two excellent presentations on the Conceptual Models for the Southern Hemisphere (CM4SH) project. The rationale behind CM4SH is that the vast majority of weather forecasting ‘wisdom’ is derived from Northern Hemisphere perspectives, through an accident of history. But understanding the weather of the Southern Hemisphere isn’t as simple as flipping everything upside down. Although the physics of the weather is clearly the same, the actual meteorological situation in Southern Hemisphere countries is different. For example, South Africa lies in the midlatitude belt like Europe does, but it sits rather closer towards the Equator, so the same weather system could have different effects. The configuration of Southern Hemisphere land masses is very different, and that leads to rather different weather behaviour.
CM4SH is a collaboration between the national meteorological services of South Africa, Argentina, Australia and Brazil. The work focused on building up a catalogue of typical meteorological situations in different regions of the Southern Hemisphere, analysing similarities and differences. The international CM4SH team used Google Drive to build a catalogue of these situations, their typical causes, behaviour and effects. Satellite imagery is obviously a major part of the catalogue, as it allows forecasters to track the flow of moisture, presence of clouds, direction and strength of winds. The resulting catalogue allows Southern Hemisphere forecasters to classify meteorological situations and quickly find out the typical effects of different systems. For example, if a forecaster sees a particular meteorological configuration, they can quickly check the catalogue for the effects of similar situations in the past, and see that they need to assess the risk of, say, flooding, in a vulnerable region.
I think projects like this reflect the power of the Internet to supercharge our science. Earlier this week I wrote about how the data from the new GPM mission were available and easily accessible within weeks. GPM is a huge international collaboration combining the resources of a whole constellation of satellites. CM4SH is a project which makes use of expertise from four national meteorological services to create an unprecedented collaborative resource for forecaster training and education, freely available. The CM4SH catalogue will grow over time and become more refined – the beauty of collaborative projects like this is that, as long as someone does a little pruning now and then, they can only ever become more useful.
EUMETSAT Post 1: Challenges and advances in satellite measurement
EUMETSAT Post 2: Socioeconomic benefits of meteorological satellites